Making the Medicines
Most Likely to Work
Related Sciences (RS) is a data science-driven drug foundry designed from the ground up to take full advantage of the last decade's extraordinary advances in biology, engineering, and machine learning. RS uses a proprietary predictive AI platform to systematically identify the best new drug creation opportunities out of millions and a novel decentralized R&D model to bring the world's top scientists together to make them. RS leverages these capabilities to build an evergreen pipeline of new medicines with exceptional clinical and economic promise.
Evidence-Driven Strategy
To prioritize RS investment into the new medicines with the greatest chances of success, RS seeks to discover data science-driven insights into big risk-reward questions like:
What Factors Increase a Drug's Odds of Success?
A biotech’s early decision of which drug target(s) to pursue for which disease(s) can influence its future chances of success and failure by 200-500%+. Systematic analysis of biomedicine's historical outcomes leveraging machine learning can elucidate factors that can prospectively enhance each drug program's built-in odds of clinical and economic success ("Money Ball for biotech")? 1, 2, 5
Which Strategies and Models Enhance Risk-Reward?
RS spent years studying biotech's top operators and investors to identify new strategies and operating models that can enhance scientific quality and tech-enablement while enhancing cost and capital efficiency.
Are the Best Discoveries Made into Medicines?
Analysis of all current biomedical knowledge on the RS data platform reveals the surprising finding that ~75% of all target-disease opportunities with the strongest evidence of links have never been tried: 20,000+ opportunities are just waiting to be made into promising new medicines. 4
A Golden Era
for Drug Creation
Parallel revolutions in data science, biology, therapeutic modalities, discovery technologies, and the capabilities of the global research services sector, compound the probability of transformative new discoveries and position drug creation for decades of high value creation.
Data, Machine Learning, and AI
Approximately 250 million target-disease pairs collectively represent the "cures solution space" to treat all known diseases. Selecting which target-disease opportunities to prioritize is the hard part.
Fortunately, as human clinical and genetics datasets continue to grow rapidly each year, the strongest potential disease targets are continually revealed. And as large language and machine learning models become increasingly capable, it becomes possible to apply data science to systematically evaluate all lines of evidence in tandem to rank and make quantitative predictions about the very best new drug creation opportunities out of millions.
Multi-Technology Discovery
Over the last decade, 10+ new therapeutic modalities have been invented, refined, and clinically validated, significantly broadening the drug-ability of historically challenging targets and unlocking superior new drug safety and efficacy properties.
In parallel, revolutions in drug discovery technologies--AI-enabled structural prediction, in silico screening and design, high-throughput robotics, and advanced new compound libraries and screening methods--further increase success rates, speed, and efficiency.
When combined, these extraordinary advances open up a golden era for drug discovery in which new, better drugs can be created for 5,000+ untreated or underserved disease populations.
Decentralized Science
Science is inherently global, and yet most US biotech remains local, with ~60-70% of US biotech VC invested in just 2 states.
Fortunately, the proliferation of remote collaboration tools unlocks extraordinary opportunities to reinvent traditional scientific staffing models to overcome geographic constraints and engage with the best scientific talent wherever it may be found.
Large, fully virtual teams can now work together seamlessly across countries, research disciplines, vendors, and both academia and industry, collaboratively crafting world-class R&D strategies to improve quality and remove scientific blind spots.
Hub-and-Spoke R&D
Over the last decade, as large Pharma companies shuttered their early-stage research centers, a flood of talented R&D scientists made their way into global contract research organizations (CROs) dramatically expanding their offerings, capabilities, and scale.
As a result, a flourishing new virtual R&D economy now offers democratized, on-demand access to a wide range of highly specialized R&D capabilities, enabling biotech companies to 1) take better advantage of a broader swathe of new technologies to increase success rates, drug quality, pace, and efficiency; and 2) convert fixed overhead into fully variable expenses under lean, specialized management teams, eliminating the 40-60% of capex historically focused on building out labs and hiring generalists.

A Fully-Integrated
Drug Foundry
Instead of betting on any one technology, target, or disease, the RS operating and investment model is predicated on integration as the innovation, combining modular capabilities that enable our drug creation efforts to benefit from superior scientific quality, technology enablement, capital efficiency, and scalability.

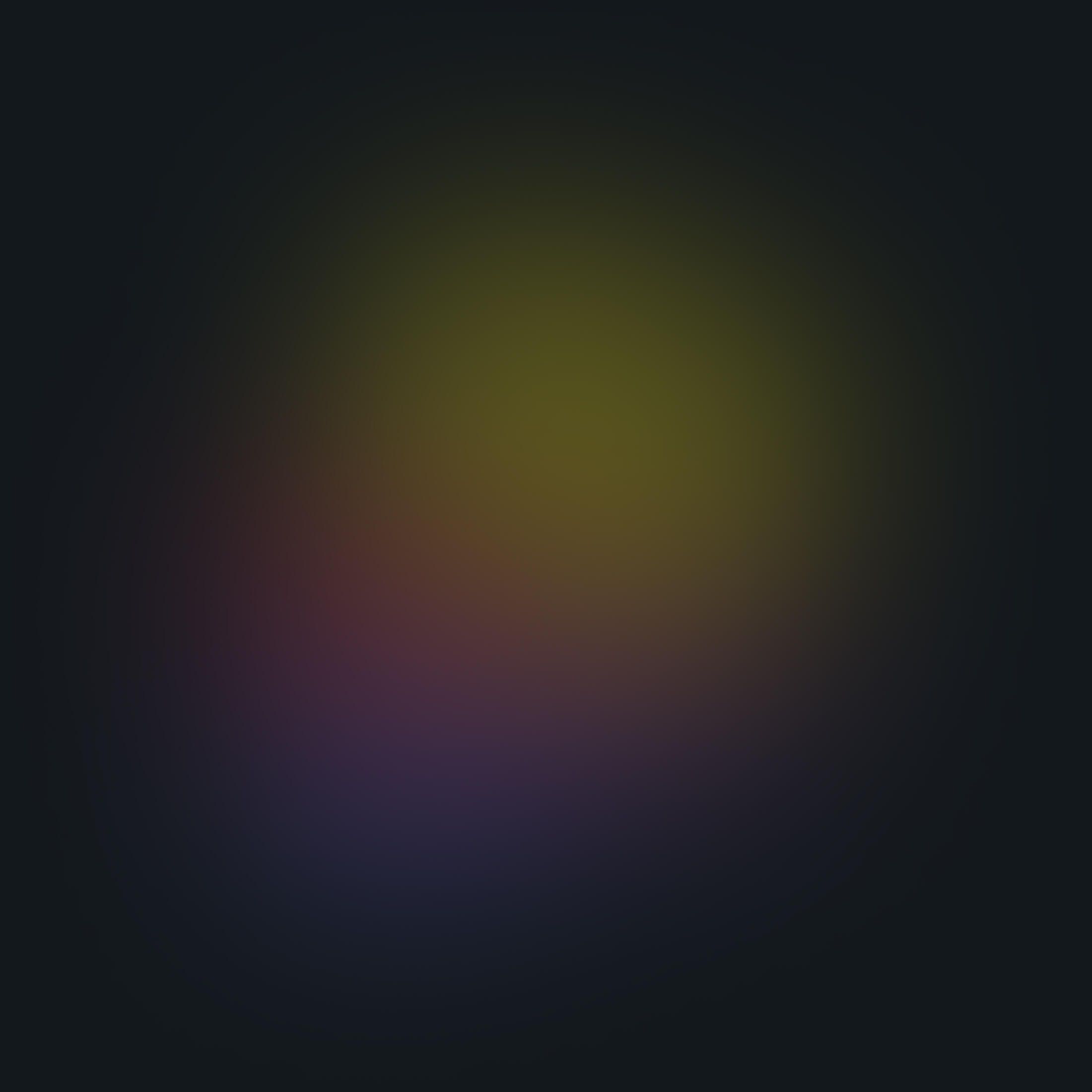

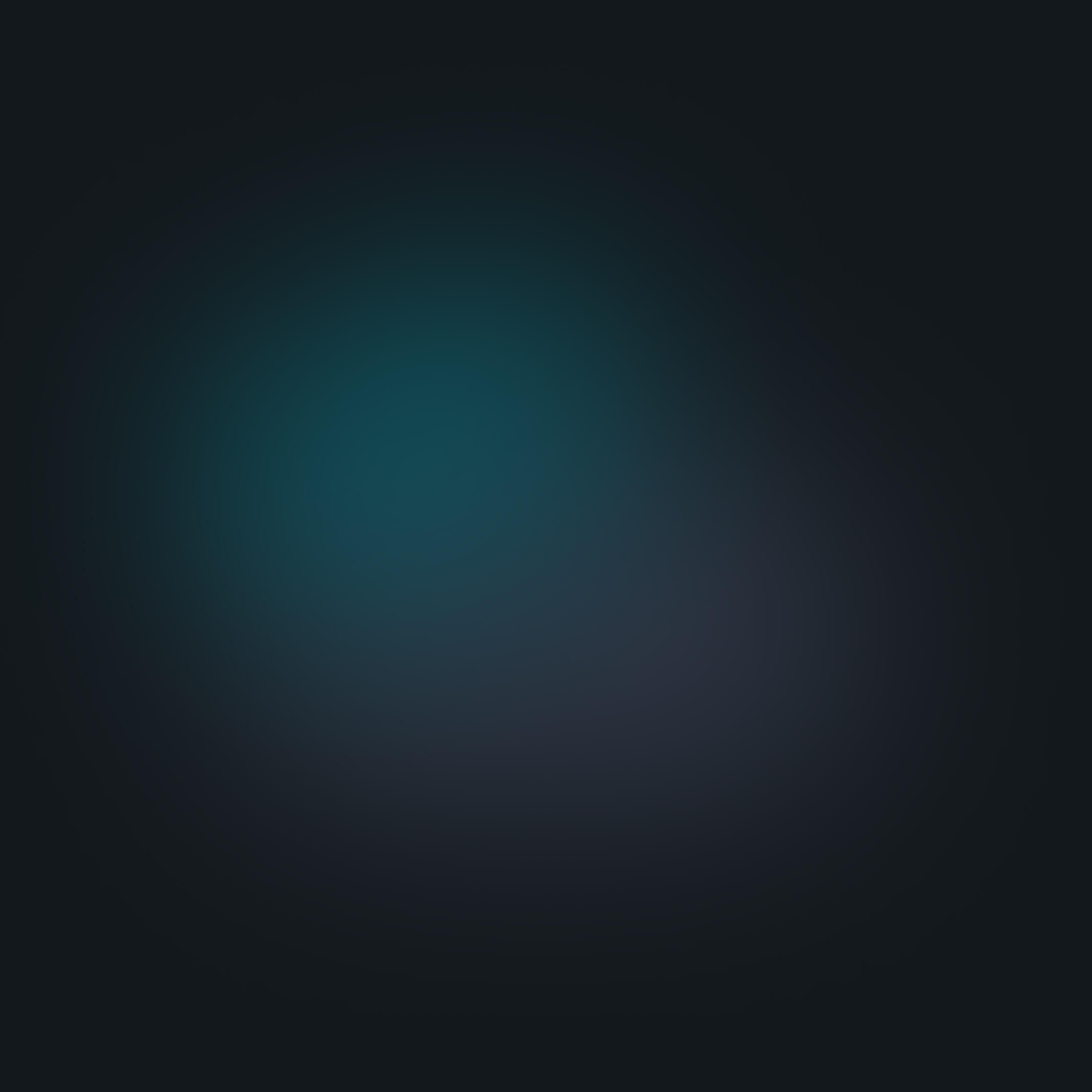

FacetsTM️ AI/ML Platform
Must 90%+ of drugs inevitably fail? Or can programs with far higher chances of success be identified prospectively? RS FacetsTM is a proprietary machine learning platform designed to ingest all activities in global biomedicine and systematically predict the best new drug discovery opportunities for every disease on an unbiased, quantified, and explainable basis.
New Datasets & ML Models
3 Layer Architecture:
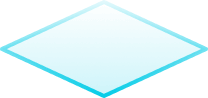
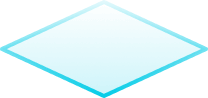
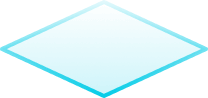
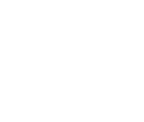
All of Biomedicine in One Dataset
RS curates, cleans, and integrates 70+ public, private, and RS-proprietary data sets covering virtually all historical activities and outcomes in global biomedicine, from early research through to value creation, into a single large time-resolved relational database--the RS Biomedical Atlas.
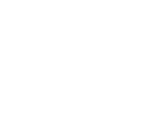
Quantitative Opportunity Ranking
Leveraging its integrated datasets, RS scores each of ~250 million possible target-disease drug discovery opportunities across 100s of proprietary metrics to systematically assess each potential program's relative risk-reward profile using a system designed to recapitulate most considerations seasoned drug discovery R&D leaders and investors use to evaluate each drug program.
Predictive AI/ML Models
Combining these two proprietary layers, RS can build proprietary machine learning models designed to predict each program's probability of clinical success and economic value creation, and to globally rank millions of drug discovery opportunities to identify the very best ones for each disease.
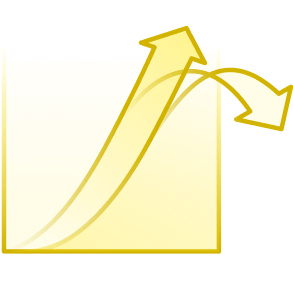
Drug Creation, Systematized
On a risk-adjusted basis, preclinical drug discovery generates among the highest risk-adjusted value of any stage in drug development.2 Instead of rebuilding overhead in each new company, RS centralizes and systematizes world-class capabilities to efficiently discover valuable new drugs, and invests in unique portfolios of top-ranking programs.
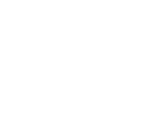
Lean Processes, Specialized Teams
RS drug discovery is led by specialized senior leaders and world-class technical advisors, following unique process management strategies. By looking at successful drug discovery as an interdisciplinary, functional "assembly line", RS enables new drug discovery programs to initiated without rebuilding the core backbone expertise architecture with each new portfolio.

Data-Designed Portfolios
RS builds and invests in a unique portfolio-based asset class called "constellations". Each RS constellation represents a themed portfolio of 5-15 top-ranking drug discovery opportunities in an ascendant area of research and commercial interest, offering optimal risk-reward characteristics at the level of individual programs matched to portfolio-level risk diversification and R&D synergies.
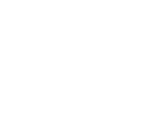
Flexible Exit Strategies
Earlier exits at lower valuations but with significantly smaller risks of failure, timelines, and capital requirements can offer enhanced overall risk-adjusted expected returns. RS optimizes its program selection, team design, and business development strategies to increase the possibilities of early acquisition interest in completed preclinical drugs while retaining full optionality to progress into the clinic.
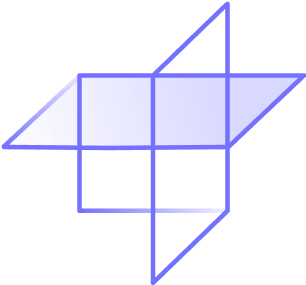
Decentralized Team Science
RS combines decentralized team science with fully virtual operations and shared back-office to enable world-class science without geographic constraint while reducing its R&D cost structures by 40%+.

Big Global Multi-disciplinary Teams
RS assembles field-leading academic scientists and clinicians from around the world into big, collaborative teams tailored to the unique expertise needs of each individual drug program. This “fractional CSO/CMO” model replaces the traditional combination of full-time generalists and passive quarterly “Scientific Advisory Board” members, with a more engaging team science model that yields both superior science and dramatically improved cost efficiency and flexibility.
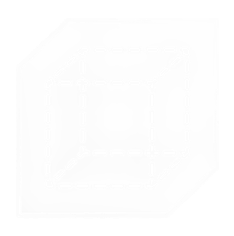
Fully Virtual + Shared Back-Office
RS drug programs operate leanly over a shared suite of RS capability platforms that provide access to the specialized interdisciplinary staff, labs, and technologies needed for world-class drug discovery without requiring any incremental overhead investments. By eliminating biotech restaffing requirements, capital-intensive lab build-outs, and geographic constraints, RS can build companies that leverage the best talent and capabilities wherever they may live while dramatically reducing fixed overhead and non-R&D uses of capital.

Tax-Efficient Corporate Structure
The RS corporate structure is designed as a series of pass-through layers, enabling the flexibility to sell individual drug programs, bundles, or whole company portfolios to be sold with single-layer corporate tax and without having to sell operations.

Hub-and-Spoke R&D
RS continually curates and partners with the world's most advanced drug discovery service providers to provide centralized access to the most advanced R&D capabilities under highly preferred cost structures and well-aligned risk-sharing business deals.

Risk-Sharing R&D Partnerships
RS curates and builds long-term strategic partnerships with top research services organizations, discovery platforms, and technology companies around the world to maximize its chances of finding extraordinary new chemical matter, at preferred cost structures, and with uniquely aligned incentives aligned for quality and success. RS currently works with 8 major R&D partners, including drug discovery leader, Evotec, which provides RS with access to its global network of cutting-edge laboratories, multi-modality screening capabilities, and 1,000’s of expert drug discovery scientists.
Multi-Technology Enablement
Drug discovery technologies continually advance as new high throughput screening and robotics systems, compound libraries, in vitro and in vivo models, reagents, assays, and analysis methods, and in silico/AI drug design tools all improve and compound on each others' benefits. RS continually curates and centralizes access to the latest discovery technologies to maximize its companies' end-to-end discovery efficiency, pace, quality, and overall odds of success.
Multi-Modality Discovery
Different modalities offer unique advantages for different types of targets. To take maximal advantage of these important new capabilities in a modality-agnostic manner, RS curates and partners with specialist vendors, builds teams of technical advisors, and often pursues parallel approaches for each target. At current, RS can make 10+ different types of medicine.
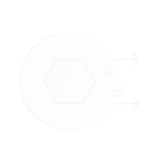
Data-Driven Indication Strategy
Leveraging its Facets™ data platform, clinical landscape mapping, and working with integrated teams of top clinician-scientists, hospitals, and academic research groups, RS systematically identifies the best disease opportunities for each program, crafting “pipelines-in-a-product” that maximize predicted expected odds of clinical success, patient impact, and economic value creation.
Team
RS is led by a multi-disciplinary team of drug discovery, data science, and venture capital veterans who have founded, built, and led biotech companies worth billions, and is backed by a luminary group of investors.
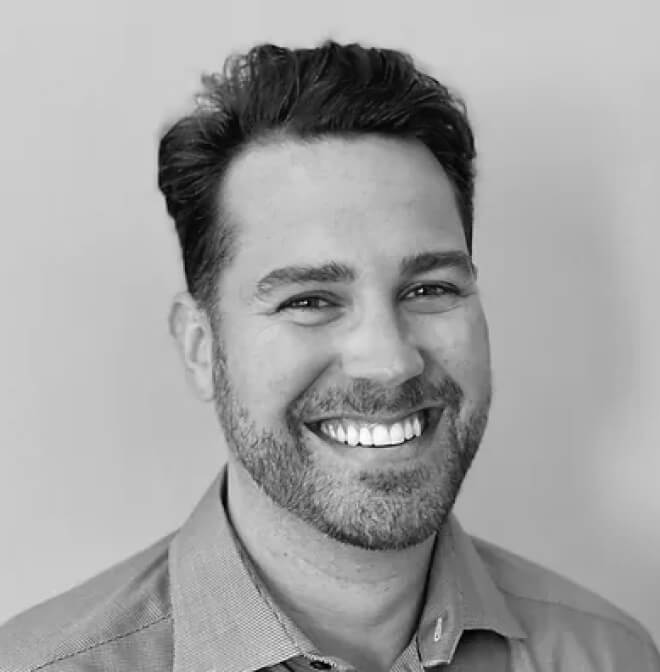
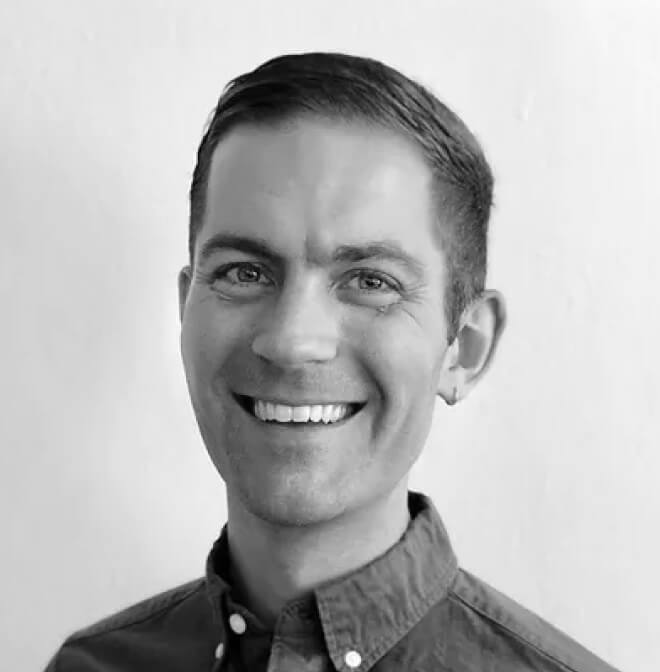
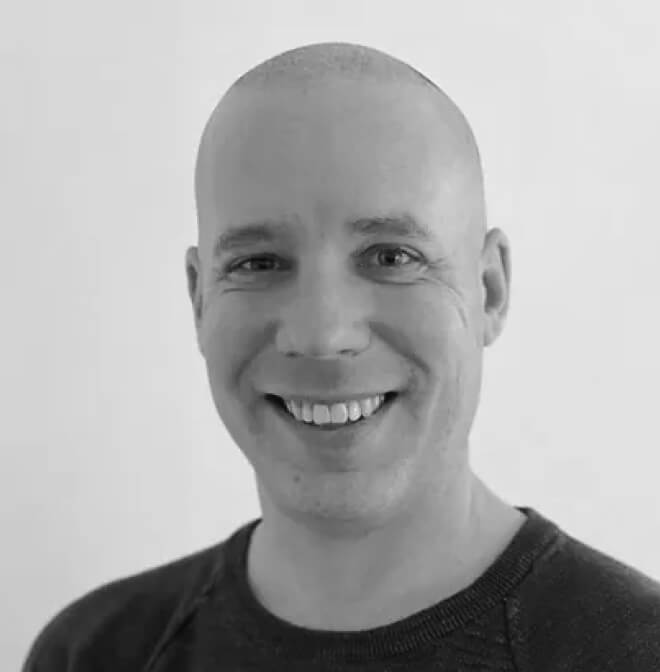
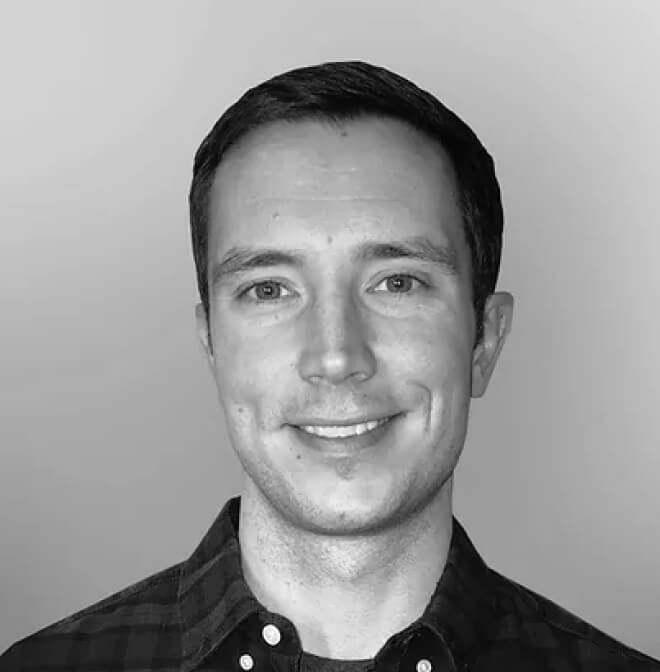
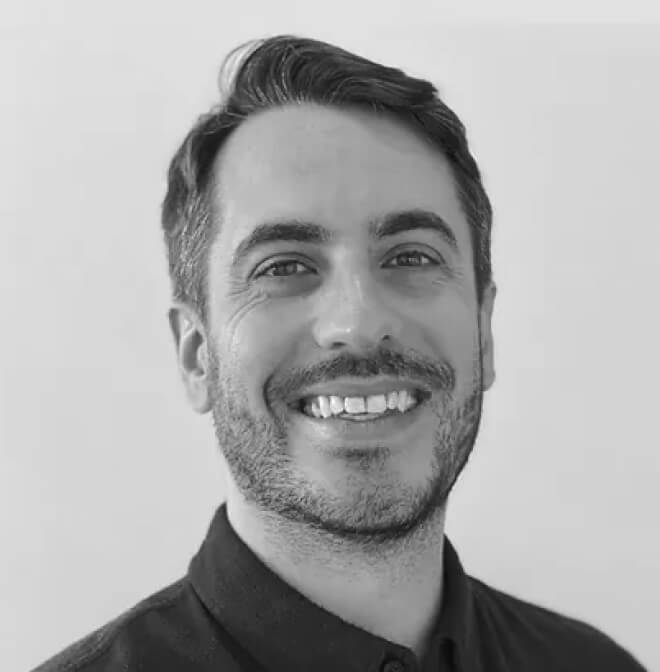

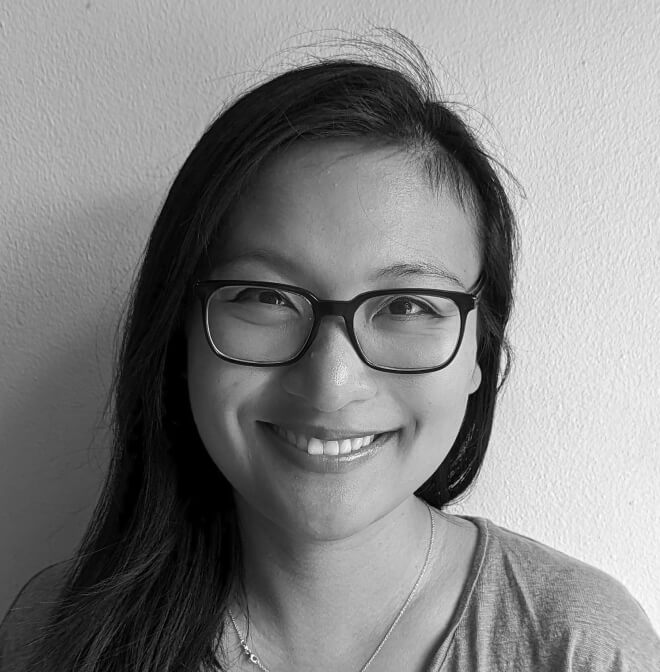
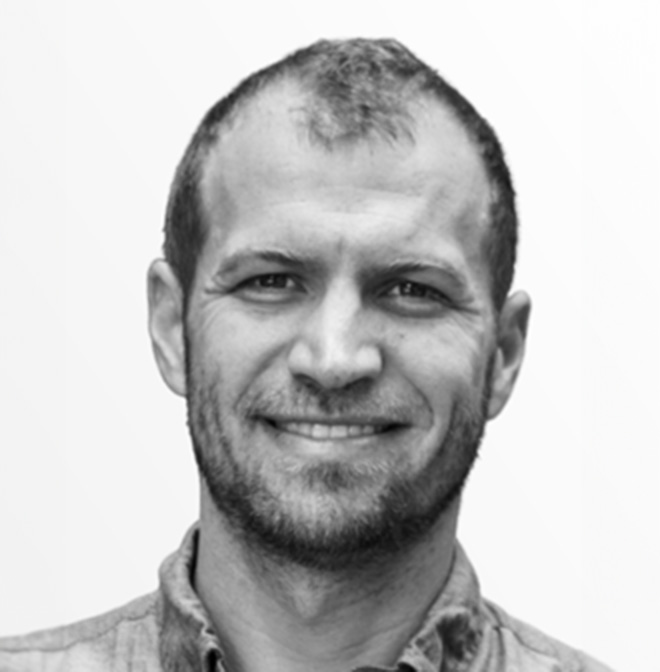

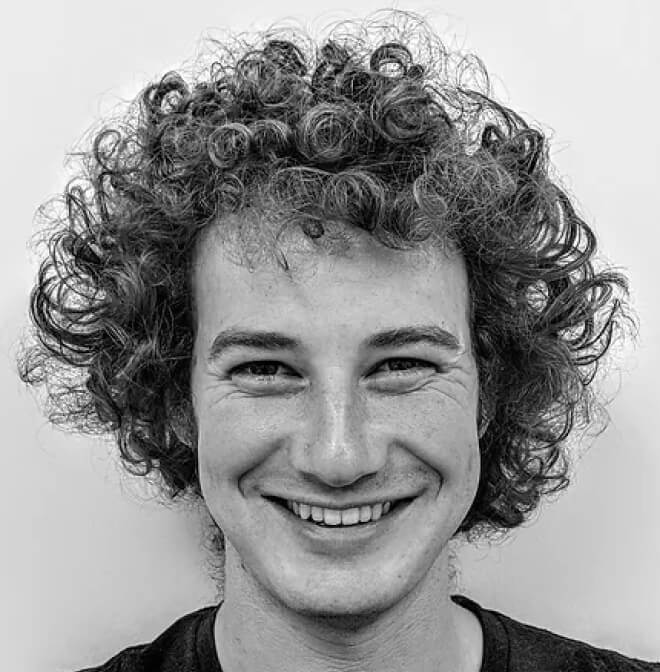




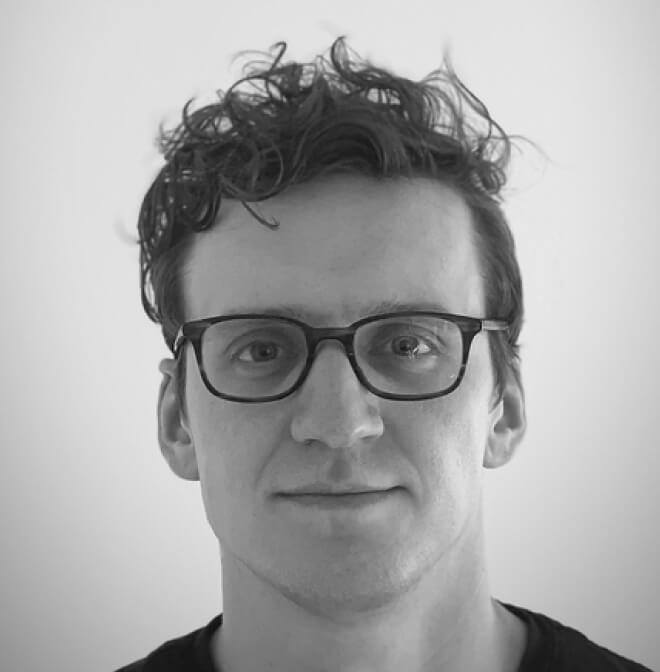
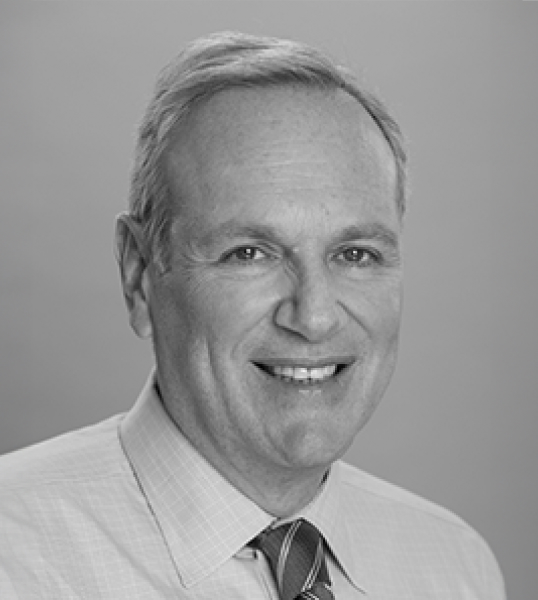
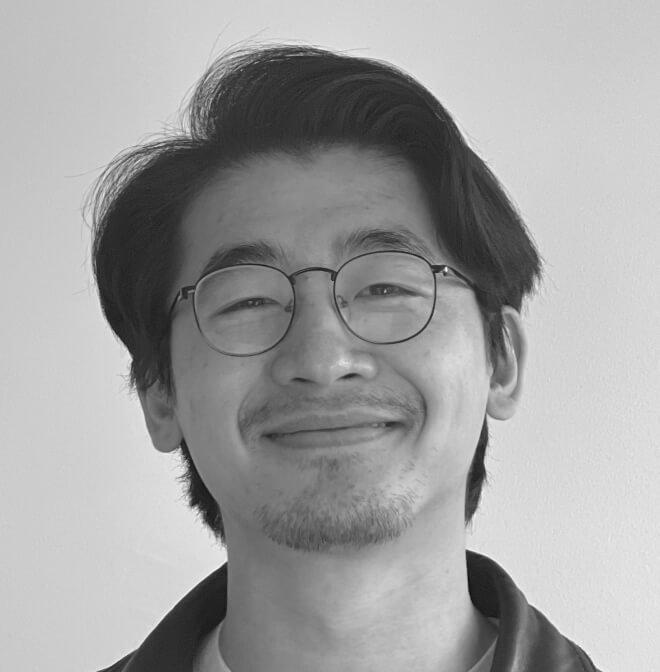

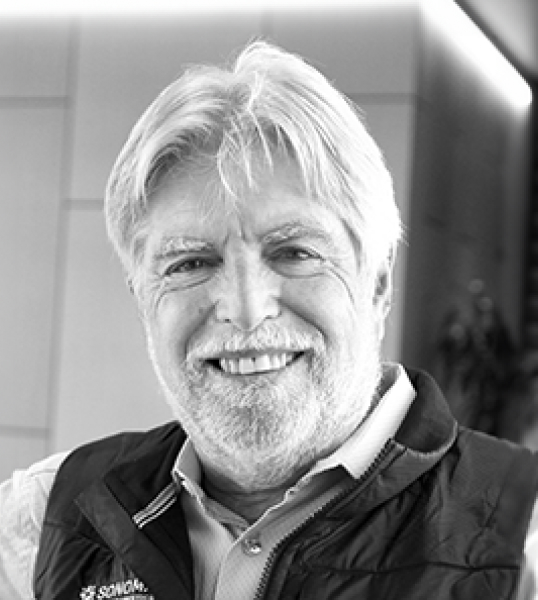
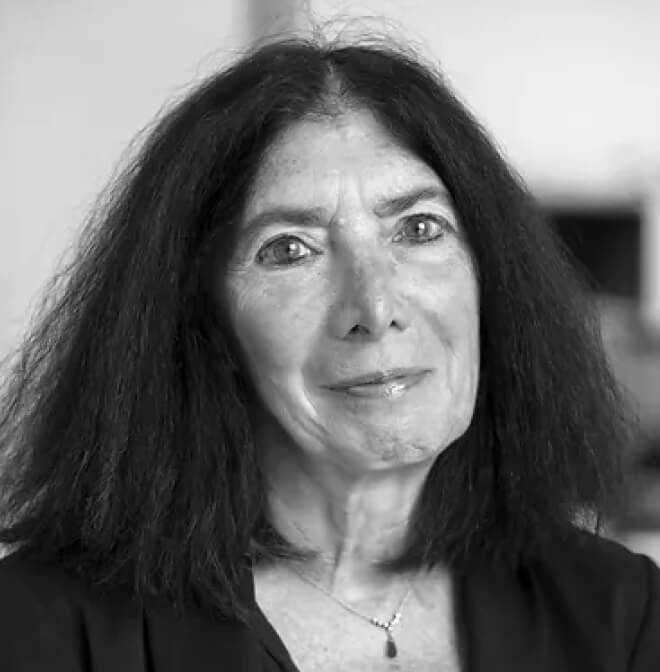




Pipeline
RS builds “constellations” -- data-designed portfolios of top-ranking drug programs in ascendant areas of commercial interest, led by decentralized teams of field-leading scientists tailored to the unique needs of each program and research area.
Vision
Building a new model to improve drug discovery efficiency and success rates benefits all of biomedicine's key stakeholders:
Patients
A greater number of transformational new medicines for virtually all types of disease are discovered sooner and successfully brought to market more often.
Researchers
Breakthrough research is successfully translated into medicines more often, and our new model enables leading researchers to contribute to biotech R&D without needing to leave academia.
Entrepreneurs
Biotech company failure is significantly reduced, driving significantly lower job turnover and training a deeper bench of capable biotech operators for the next generation of drugs.
Pharma
Supply of high quality, clinic-ready drugs is dramatically increased and made available at acquisition prices that both incentivize biotech and are sustainable for pharma’s own economic models.
Investors
Better biotech risk-reward drives a much greater proportion of investor wins broadening interest among institutional investors to fund future biotech innovations.